
Data content
rSNPBase includes rSNPs, LD-proxies of rSNPs, and genes that are potentially regulated by rSNPs. Experimentally supported regulatory elements were collected and utilized to annotate the regulatory feature of rSNPs. Regulation-related spatio-temporal information and experimental eQTL evidence are used as data labels for involoved SNPs. The data for rSNPBase(as of August 1, 2013) are shown in Table 1.
a: A rSNP may be involved in multiple types of regulation. b: In rSNPBase, SNPs (both rSNP and non-rSNP) in strong LD (r2 > 0.8) with a rSNP are defined as LD-proxies. Here we only count the number of non-rSNPs of the LD proxies.
Data processing
Genome-wide human SNPs and genes were filtered and mapped by experimentally validated regulatory elements, which are involved in four types of regulation (proximal and distal transcriptional regulation and RBP-mediated and miRNA-mediated post-transcriptional regulation). As shown in Figure 1, rSNPBase hosts rSNPs that are within regulatory elements. Element-regulated genes were also analyzed and hosted as genes potentially regulated by rSNPs. For each rSNP, SNPs (both rSNP and non-rSNP) in strong LD (r2> 0.8) are located with reference to data from the HapMap project and 1000 Genome project. Finally, spatio-temporal labels and eQTL labels are generated and included on all SNPs.
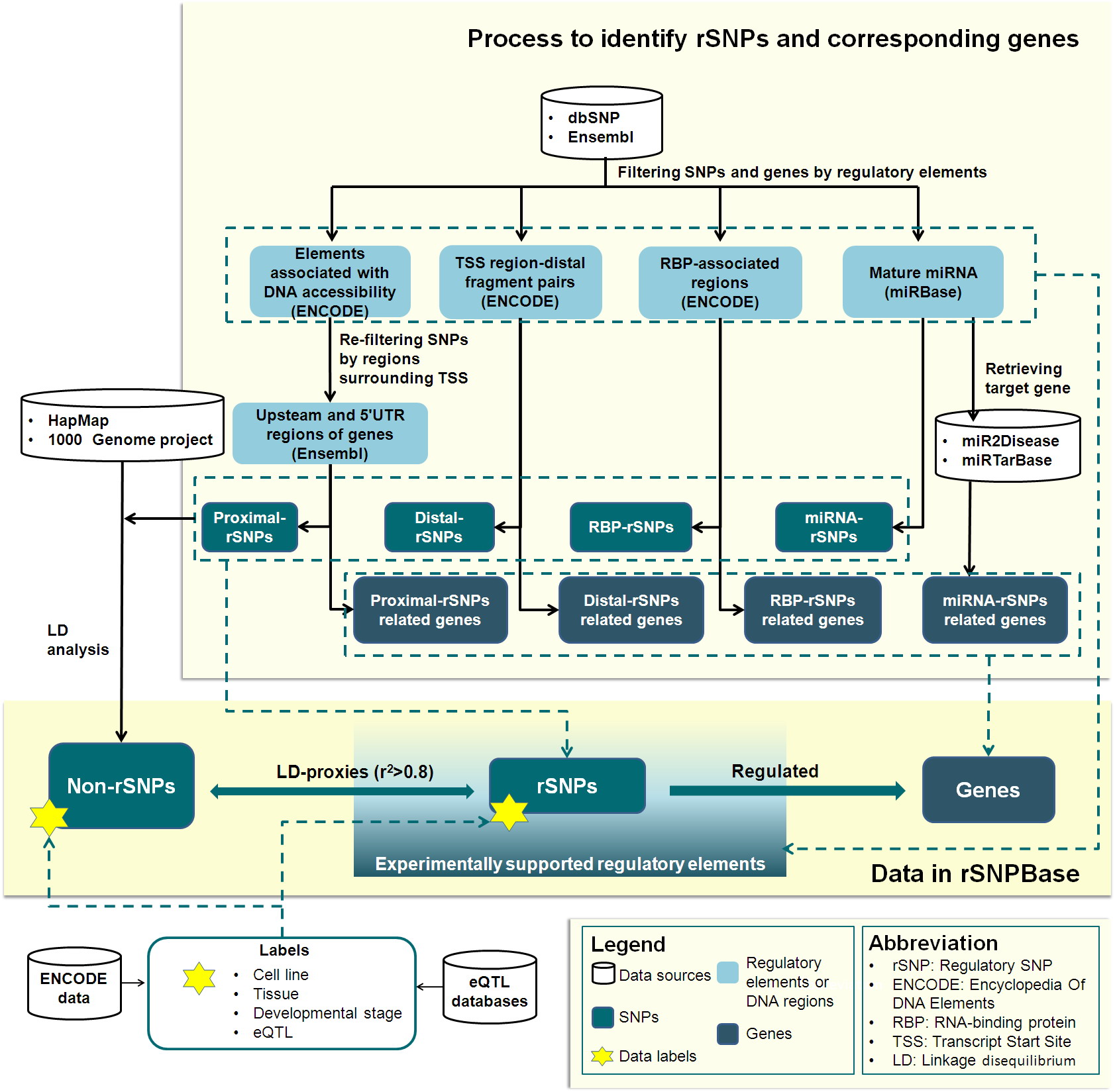
Figure 1 Data processing and data content of rSNPBase.
Generating regulatory elements involved in different types of regulation
Processed ENCODE production data that are associated with chromatin accessibility (including open chromatin, histone marked regions, CpG islands, and transcription factor binding sites), chromatin interactions, and RBPs were downloaded from the UCSC Genome Browser (hg 19)(1)(http://genome.ucsc.edu/ENCODE/downloads.html) to generate experimentally validated regulatory elements involved in proximal and distal transcriptional regulation and RBP-mediated post-transcriptional regulation (the ENCODE data that are utilized in rSNPBase are shown in Table 2). The same type of data is integrated, and redundant data are pruned. Specific for histone modification data, only regions marked by active-associated histones (H3K4me1, H3K4me2, H3K4me3, H3K9ac, H3K27ac, H3K36me3, H3K79me2, H4K20me1, and H3K9me1)(2-4)are included in rSNPBase. Mature miRNAs were collected from miRBase (release 20)(5)as regulatory elements involved in miRNA-mediated post-transcriptional regulation.
Analyzing rSNPs and corresponding genes involved in different types of regulation
Human SNPs from dbSNP (build 137) (6)are filtered using experimentally validated regulatory elements based on the genomic location to identify rSNPs. According to the involved regulation types, element-filtered SNPs are defined as proximal transcriptional rSNPs (proximal-rSNPs), distal transcriptional rSNPs (distal-rSNPs), RBP-mediated post-transcriptional rSNPs (RBP-rSNPs), and miRNA-mediated post-transcriptional rSNPs (miRNA-rSNPs),all of which are termed rSNPs in rSNPBase. Human genes from Ensembl (GRCh37. P11)(7)are mapped by regulatory elements or analyzed with reference to experimentally supported databases to identify genes corresponding with rSNPs. Proximal transcriptional regulation is related to elements associated with DNA accessibility, and this type of regulation is largely dependent on the genomic proximity of the elements and transcript start site (TSS). Therefore, SNPs filtered by relevant elements are re-filtered by upstream and 5'UTR regions of genes.The final double-filtered SNPs are defined as proximal-rSNPs, and their corresponding genes are identified with reference to their consequence types, which are cataloged by Ensemblbl(7). Distal transcriptional regulation-related elements are analyzed from the ENCODE data of chromatin interactions. This type of data provides interacted TSS-fragment pairs that are distant in sequence but relatively close in space. For each TSS-fragment pair, the distal-rSNPs are identified from the distal fragment, and their corresponding genes are identified from the TSSs located in the paired region. Sometimes both interacting regions contain TSSs,rSNPs are then generated from both regions correspondingly. DNA elements related to RBP-mediated post-transcriptional regulation are mapped from RBP-associated RNA sequences generated by ENCODE. SNPs falling within these regulatory elements are defined as RBP-rSNPs. Genes that are mapped by RBP-associated RNA sequences correspond with this type of rSNP. SNPs within mature miRNAs recorded by miRBase are defined as miRNA-rSNPs and correspond with miRNA-targeted genes, which are obtained from the experimentally supported miRNA-targeted gene database miR2Disease(8) and miRTarBase(9).
Analyzing LD proxies
Because of the genetic correlation between nearby SNPs, it will miss information to do functional analysis on single SNP. To extend the regulatory feature of a single SNP to its correlating genome structure, LD correlations between SNPs are analyzed, and the set of SNPs that are in strong LD (r2>0.8) with the rSNPs are defined as LD-proxies of rSNPs. The LD data are compiled from both merged HapMap phases I+II+III genotype data for markers that are up to 200 kb apart(10) and integrated 1000-genomes phase I release data(11,12), which are downloaded from the International HapMap Consortium and MaCH(13). Data from all populations that the two projects are involvedin are all utilized to perform LD analyses.
Adding data labels
Due to the importance of eQTL evidence for deciphering gene regulation and the spatio-temporal specificity of gene regulation, rSNPBase provides eQTL labels and spatio-temporal labels for the included SNPs. eQTL attributes are collected from experimentally supported eQTL databases (14-16) and eQTL browser (http://eqtl.uchicago.edu/cgi-bin/gbrowse/eqtl/) (17-23)to provide association labels for SNPs.Tissue and developmental stage information are labeled according to cell type, from which regulatory elements are identified.
Reference:
1. Rosenbloom, K.R., Sloan, C.A., Malladi, V.S., Dreszer, T.R., Learned, K., Kirkup, V.M., Wong, M.C., Maddren, M., Fang, R., Heitner, S.G. et al. (2013) ENCODE data in the UCSC Genome Browser: year 5 update. Nucleic acids research, 41, D56-63.
2. Barski, A., Cuddapah, S., Cui, K., Roh, T.Y., Schones, D.E., Wang, Z., Wei, G., Chepelev, I. and Zhao, K. (2007) High-resolution profiling of histone methylations in the human genome. Cell, 129, 823-837.
3. Creyghton, M.P., Cheng, A.W., Welstead, G.G., Kooistra, T., Carey, B.W., Steine, E.J., Hanna, J., Lodato, M.A., Frampton, G.M., Sharp, P.A. et al. (2010) Histone H3K27ac separates active from poised enhancers and predicts developmental state. Proceedings of the National Academy of Sciences of the United States of America, 107, 21931-21936.
4. Liang, G., Lin, J.C., Wei, V., Yoo, C., Cheng, J.C., Nguyen, C.T., Weisenberger, D.J., Egger, G., Takai, D., Gonzales, F.A. et al. (2004) Distinct localization of histone H3 acetylation and H3-K4 methylation to the transcription start sites in the human genome. Proceedings of the National Academy of Sciences of the United States of America, 101, 7357-7362.
5. Kozomara, A. and Griffiths-Jones, S. (2011) miRBase: integrating microRNA annotation and deep-sequencing data. Nucleic acids research, 39, D152-157.
6. Sherry, S.T., Ward, M.H., Kholodov, M., Baker, J., Phan, L., Smigielski, E.M. and Sirotkin, K. (2001) dbSNP: the NCBI database of genetic variation. Nucleic acids research, 29, 308-311.
7. Flicek, P., Ahmed, I., Amode, M.R., Barrell, D., Beal, K., Brent, S., Carvalho-Silva, D., Clapham, P., Coates, G., Fairley, S. et al. (2013) Ensembl 2013. Nucleic acids research, 41, D48-55.
8. Jiang, Q., Wang, Y., Hao, Y., Juan, L., Teng, M., Zhang, X., Li, M., Wang, G. and Liu, Y. (2009) miR2Disease: a manually curated database for microRNA deregulation in human disease. Nucleic acids research, 37, D98-104.
9. Hsu, S.D., Lin, F.M., Wu, W.Y., Liang, C., Huang, W.C., Chan, W.L., Tsai, W.T., Chen, G.Z., Lee, C.J., Chiu, C.M. et al. (2011) miRTarBase: a database curates experimentally validated microRNA-target interactions. Nucleic acids research, 39, D163-169.
10. Altshuler, D.M., Gibbs, R.A., Peltonen, L., Dermitzakis, E., Schaffner, S.F., Yu, F., Bonnen, P.E., de Bakker, P.I., Deloukas, P., Gabriel, S.B. et al. (2010) Integrating common and rare genetic variation in diverse human populations. Nature, 467, 52-58.
11. Abecasis, G.R., Altshuler, D., Auton, A., Brooks, L.D., Durbin, R.M., Gibbs, R.A., Hurles, M.E. and McVean, G.A. (2010) A map of human genome variation from population-scale sequencing. Nature, 467, 1061-1073.
12. Patterson, K. (2011) 1000 genomes: a world of variation. Circulation research, 108, 534-536.
13. Li, Y., Willer, C.J., Ding, J., Scheet, P. and Abecasis, G.R. (2010) MaCH: using sequence and genotype data to estimate haplotypes and unobserved genotypes. Genetic epidemiology, 34, 816-834.
14. Xia, K., Shabalin, A.A., Huang, S., Madar, V., Zhou, Y.H., Wang, W., Zou, F., Sun, W., Sullivan, P.F. and Wright, F.A. (2012) seeQTL: a searchable database for human eQTLs. Bioinformatics, 28, 451-452.
15. Gamazon, E.R., Zhang, W., Konkashbaev, A., Duan, S., Kistner, E.O., Nicolae, D.L., Dolan, M.E. and Cox, N.J. (2010) SCAN: SNP and copy number annotation. Bioinformatics, 26, 259-262.
16. (2013) The Genotype-Tissue Expression (GTEx) project. Nature genetics, 45, 580-585.
17. Schadt, E.E., Molony, C., Chudin, E., Hao, K., Yang, X., Lum, P.Y., Kasarskis, A., Zhang, B., Wang, S., Suver, C. et al. (2008) Mapping the genetic architecture of gene expression in human liver. PLoS biology, 6, e107.
18. Myers, A.J., Gibbs, J.R., Webster, J.A., Rohrer, K., Zhao, A., Marlowe, L., Kaleem, M., Leung, D., Bryden, L., Nath, P. et al. (2007) A survey of genetic human cortical gene expression. Nature genetics, 39, 1494-1499.
19. Stranger, B.E., Nica, A.C., Forrest, M.S., Dimas, A., Bird, C.P., Beazley, C., Ingle, C.E., Dunning, M., Flicek, P., Koller, D. et al. (2007) Population genomics of human gene expression. Nature genetics, 39, 1217-1224.
20. Veyrieras, J.B., Kudaravalli, S., Kim, S.Y., Dermitzakis, E.T., Gilad, Y., Stephens, M. and Pritchard, J.K. (2008) High-resolution mapping of expression-QTLs yields insight into human gene regulation. PLoS genetics, 4, e1000214.
21. Pickrell, J.K., Marioni, J.C., Pai, A.A., Degner, J.F., Engelhardt, B.E., Nkadori, E., Veyrieras, J.B., Stephens, M., Gilad, Y. and Pritchard, J.K. (2010) Understanding mechanisms underlying human gene expression variation with RNA sequencing. Nature, 464, 768-772.
22. Montgomery, S.B., Sammeth, M., Gutierrez-Arcelus, M., Lach, R.P., Ingle, C., Nisbett, J., Guigo, R. and Dermitzakis, E.T. (2010) Transcriptome genetics using second generation sequencing in a Caucasian population. Nature, 464, 773-777.
23. Zeller, T., Wild, P., Szymczak, S., Rotival, M., Schillert, A., Castagne, R., Maouche, S., Germain, M., Lackner, K., Rossmann, H. et al. (2010) Genetics and beyond--the transcriptome of human monocytes and disease susceptibility. PloS one, 5, e10693.